Zhang Lab of Artificial Intelligence in Medicine
Division of Artificial Intelligence in Medicine at Cedars-Sinai. 6500 Wilshire Blvd, Los Angeles, CA 90048.
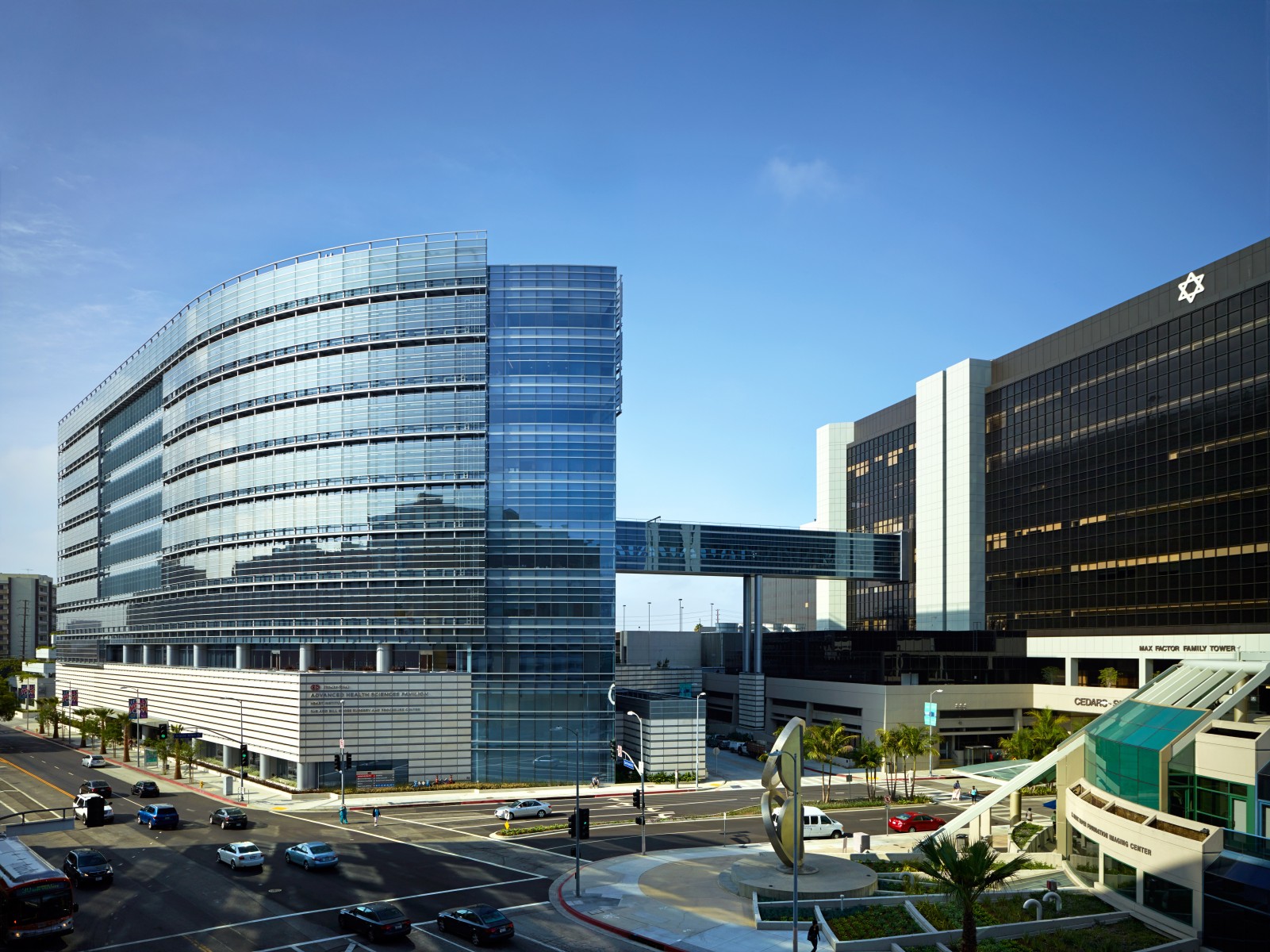
Welcome to the Zhang Lab of Artificial Intelligence in Medicine!
Our lab uses statistical learning and deep learning as the unifying language to communicate across research areas in computational biology. Currently the primary research interests include:
-
Automated Deep Learning (AutoDL)-powered Interpretation of Genetic Variations
We develop AutoDL techniques to accommodate the rapid biotechnology development with modern deep learning. These automated, data-driven models help us understand the causal effects of genetic variations in various contexts, including epigenetics, transcription, genome editing, and in tumors. In the long term, we hope our methods will democratize deep learning and thereby accelerate scientific discoveries in biomedicine.
-
Post-transcriptional and Transcriptional Regulatory Network
We also study the molecular mechanisms that mediate the genetic effects to phenotypical variations and disease prognostics. Enabled by high-throughput sequencing technologies, we characterize the variation of RNA expression, RNA splicing, RNA-protein interactions, and RNA modifications in health and disease by quantitative, statistical and deep learning approaches.
Recent News
Aug 23, 2024 | Prof Zhang Will Move And Close His Lab |
---|---|
Dec 15, 2023 | Elektrum Published At Nature Computational Science |
Nov 2, 2023 | We are honored to receive the 2023 Winnick Award! |
Oct 27, 2023 | Three Papers Accepted At Neurips23 Workshops |
Oct 19, 2023 | Emgnn Paper Accepted At Bioinformatics |